Detecting Malicious HTTP Traffic that Hides Under the Real Traffic
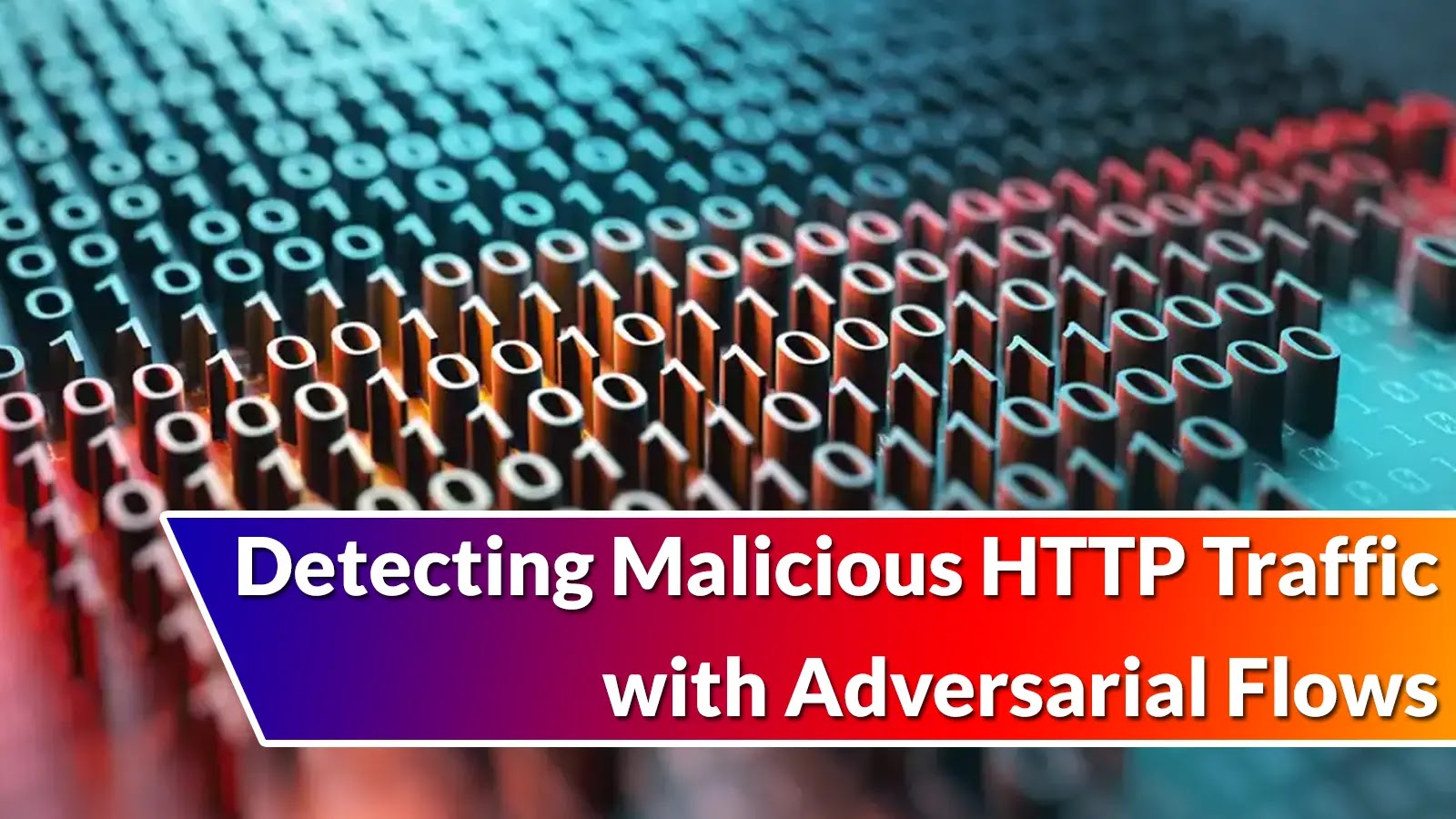
The malware generates malicious network behavior, in total hiding it in HTTP traffic to retain far from detection. So, in cyber security, detecting malicious traffic is one of many necessary complications triggered by malware.
Then all another time, apart from this, the full latest techniques primarily count on man made capabilities and out of date data, missing generalization.
The following cybersecurity researchers from their respective universities and organizations have lately unveiled how they detected malicious HTTP traffic that hides all the plan in which by means of the particular traffic:-
- Xiaochun Yun (Nationwide Laptop Community Emergency Response Technical Group/Coordination Center of China)
- Jiang Xie (Institute of Knowledge Engineering, Chinese language Academy of Sciences, and College of Cyber Security, College of Chinese language Academy of Sciences)
- Shuhao Li (Institute of Knowledge Engineering, Chinese language Academy of Sciences, Key Laboratory of Community Overview Abilities, College of Chinese language Academy of Sciences, and College of Cyber Security, College of Chinese language Academy of Sciences)
- Yongzheng Zhang (Institute of Knowledge Engineering, Chinese language Academy of Sciences, Key Laboratory of Community Overview Abilities, College of Chinese language Academy of Sciences, and College of Cyber Security, College of Chinese language Academy of Sciences)
- Peishuai Solar (Institute of Knowledge Engineering, Chinese language Academy of Sciences, and College of Cyber Security, College of Chinese language Academy of Sciences)
Deploy Developed AI-Powered Electronic mail Security Resolution
Defend your Enterprise Electronic mail from threats take care of monitoring, blocking off, modifying, phishing, anecdote takeover, industry electronic mail compromise, malware, and ransomware with Trustifi’s AI-powered electronic mail security acknowledge.
HTTP-based completely Malicious Verbal substitute Behavior
HTTP traffic carries grand of this behavior, with adversaries mimicking harmless user behavior and hiding unfavorable data within same old fields.
The similarity to threat free traffic makes the detection unprecedented, and this field additionally drives the need for superior ways.
It is wanted to reinforce detection techniques’ capability to generalize and title unknown HTTP-based completely malicious verbal substitute behavior, but it faces two most well-known challenges.
Below, we now have gotten mentioned the two challenges:-
- Feature extract
- Experimental dataset
Challenges in detecting unknown HTTP-based completely malicious behavior encompass the priority of characteristic extraction beneath adversarial prerequisites and cramped testing on minute-scale datasets, which hampers generalization capability.
Below, we now have gotten mentioned the four phases into which an HTTP-based completely malware assault could maybe maybe additionally honest additionally be divided:-
- Implantation share
- Incubation share
- Verbal substitute share
- Execution share
Effective detection of HTTP-based completely malicious behavior happens within the verbal substitute share by analyzing malware-generated traffic to title malicious interactions and discover adversaries.
Fat-duplex application layer flows contain demand and response packets with the same quintuple:-
- src_ip
- src_port
- dst_ip
- dst_port
- TCP
Apart from this, the cybersecurity researchers divide flows into packet-level and plod with the circulation-level to extract hierarchical capabilities.
The HMCD model demonstrates very honest correct detection efficiency with F1 at Ninety 9.46% within the HMCT-2020 dataset. It additionally outperforms completely different units in generalization and proper-world traffic experiments, attaining an F1 of 83.66%.
Specialists propose the HMCD-Model for detecting unknown malicious HTTP traffic, the speak of a hybrid neural network with GAN to reinforce correct traffic illustration, attaining F1 ≈ 83.66%.
HMCD improves defense in opposition to advanced attacks, with plans to compose bigger datasets and refine GAN-based completely traffic technology.
Source credit : cybersecuritynews.com