Federated Learning Based IDS – Key Challenges and Future Paths
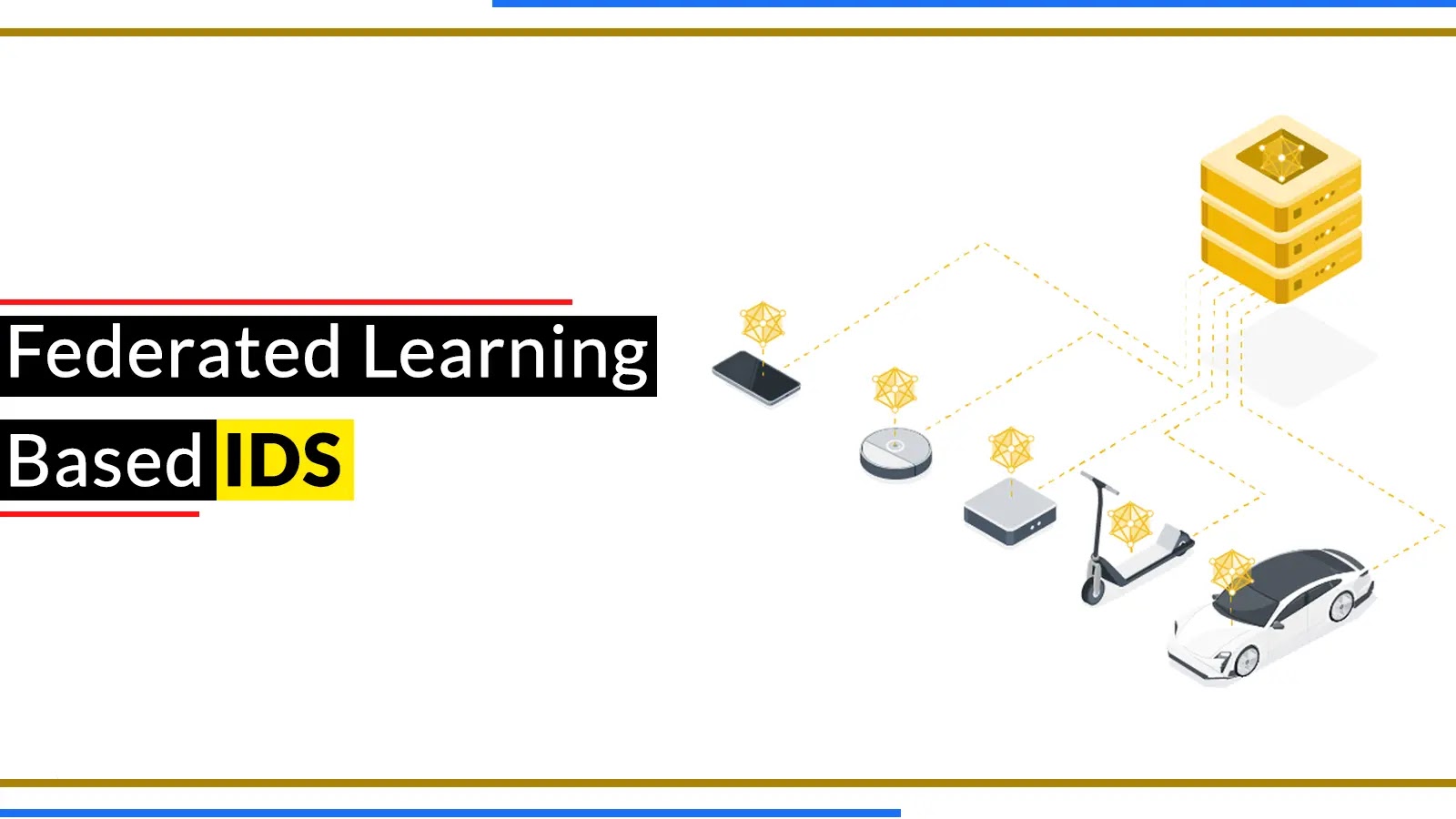
In at the present time’s digital technology, AI (Artificial Intelligence) and ML (Machine Learning) applications are surely one of many first developments.
But, with world initiatives esteem the EU AI Act and U.S. AI Strategy highlight the significance of ethical AI legislation, especially in cybersecurity.
A fable shared with Cyber Security News by a community of cybersecurity analysts consisting of Jose L. Hernandez-Ramos, Georgios Karopoulos, Efstratios Chatzoglou, Vasileios Kouliaridis, Enrique Marmol, Aurora Gonzalez-Vidal, and Georgios Kambourakis, published doubtlessly the most popular vogue within the cybersecurity arena, which is the decentralized learning intention is named “Federated Learning (FL).”
Beneath this decentralized intention, the ML units are constructed without sharing the stop nodes’ files by the utilization of a selected aggregation.
Federated Learning-Basically primarily based IDS
For the vogue of methods and applications primarily based completely on Machine Learning, this decentralized intention is necessary, and now not simplest that, but even this recent intention also helps accumulate the privacy of stop customers.
So, it sounds as if the total mechanism of this recent intention, Federated Learning (FL), is comprehensive and evolved in nature, because the protection methods can use this vogue for higher protection and protection mechanisms.
The IDS (Intrusion Detection Draw) detects the threats in IT/OT methods, and the FL-primarily based completely IDS bid links to accurate evaluate datasets.
Besides this, the preliminary evolution of IDSs used to be mainly desirous concerning the approaches which also will most definitely be signature-primarily based completely, but the recent combination of IDS with an FL-primarily based completely intention appears to be like promising and noteworthy more environment friendly than the outdated approaches.
Here below, we maintain mentioned the total datasets:-
- CIC-IDS2017
- CSE-CIC-IDS2018
- CIDDS-001
- CIDDS-002
- CIC DoS
- PUF
- TRAbID
- Unified host and network
- N-BaIoT
- Kitsune
- Bot-IoT
- AWID3
- WUSTL-IIoT
- H23Q
- IoT network intrusion dataset
- IoTID20
- IoT-23
- IoT Healthcare Security Dataset
- e IoT DoS and DDoS Assault Dataset
- CCD-INID-V1
- X-IIoTID
- Edge-IIoTset
- MQTT-IoT-IDS2020
- MQTTset
- LATAM-DDoS-IoT dataset
- UAV Assault Dataset
- CIC IoT dataset
- Intrusion Detection in CAN bus
- Ton IoT dataset
- Car Hacking
- MedBIoT
- Car Hacking: Assault & Defense Situation
- InSDN
- OTIDS
- Vehicular Reference Misbehavior Dataset (VeReMi)
In this scenario, the neural networks (NNs) extinct in IDS match the two key sides, and here below, we maintain mentioned them:-
- Enter parts
- Classes
Furthermore, the RL (Reinforcement Learning), an ML division, has agents be taught to maximize rewards by trial and mistake. Whereas it categorizes into the next methods:-
- Value-primarily based completely
- Coverage-primarily based completely
- Model-primarily based completely
Challenges Confronted
Here below, we maintain mentioned the total challenges and future trends for FL-enabled IDS approaches:-
- Security
- Privateness
- Aggregator as bottleneck
- Records heterogeneity
- Instrument heterogeneity
- Computation requirements
- Verbal substitute requirements
The enlargement of FL in IDS is clearly well-known and skyrocketed all of sudden as a result of its following key parts:-
- Decentralized
- Collaborative
- Privateness-protection
Total, it’s been concluded that this recent decentralized intention will be game-changing within the realm of cybersecurity.
Source credit : cybersecuritynews.com